Creating two-factor subplots
Let's continue looking at the student_data dataset of students in secondary school. Here, we want to answer the following question: does a student's first semester grade ("G1") tend to correlate with their final grade ("G3")?
There are many aspects of a student's life that could result in a higher or lower final grade in the class. For example, some students receive extra educational support from their school ("schoolsup") or from their family ("famsup"), which could result in higher grades. Let's try to control for these two factors by creating subplots based on whether the student received extra educational support from their school or family.
Seaborn has been imported as sns and matplotlib.pyplot has been imported as plt.
# Import Matplotlib and Seaborn import matplotlib.pyplot as plt import seaborn as sns import pandas as pd url = 'https://assets.datacamp.com/production/repositories/3996/datasets/61e08004fef1a1b02b62620e3cd2533834239c90/student-alcohol-consumption.csv' student_data = pd.read_csv(url) print(student_data)
Unnamed: 0 school sex age famsize Pstatus Medu Fedu traveltime \ 0 0 GP F 18 GT3 A 4 4 2 1 1 GP F 17 GT3 T 1 1 1 2 2 GP F 15 LE3 T 1 1 1 3 3 GP F 15 GT3 T 4 2 1 4 4 GP F 16 GT3 T 3 3 1 5 5 GP M 16 LE3 T 4 3 1 6 6 GP M 16 LE3 T 2 2 1 7 7 GP F 17 GT3 A 4 4 2 8 8 GP M 15 LE3 A 3 2 1 9 9 GP M 15 GT3 T 3 4 1 10 10 GP F 15 GT3 T 4 4 1 11 11 GP F 15 GT3 T 2 1 3 12 12 GP M 15 LE3 T 4 4 1 13 13 GP M 15 GT3 T 4 3 2 14 14 GP M 15 GT3 A 2 2 1 15 15 GP F 16 GT3 T 4 4 1 16 16 GP F 16 GT3 T 4 4 1 17 17 GP F 16 GT3 T 3 3 3 18 18 GP M 17 GT3 T 3 2 1 19 19 GP M 16 LE3 T 4 3 1 20 20 GP M 15 GT3 T 4 3 1 21 21 GP M 15 GT3 T 4 4 1 22 22 GP M 16 LE3 T 4 2 1 23 23 GP M 16 LE3 T 2 2 2 24 24 GP F 15 GT3 T 2 4 1 25 25 GP F 16 GT3 T 2 2 1 26 26 GP M 15 GT3 T 2 2 1 27 27 GP M 15 GT3 T 4 2 1 28 28 GP M 16 LE3 A 3 4 1 29 29 GP M 16 GT3 T 4 4 1 .. ... ... .. ... ... ... ... ... ... 365 365 MS M 18 GT3 T 1 3 2 366 366 MS M 18 LE3 T 4 4 2 367 367 MS F 17 GT3 T 1 1 3 368 368 MS F 18 GT3 T 2 3 2 369 369 MS F 18 GT3 T 4 4 3 370 370 MS F 19 LE3 T 3 2 2 371 371 MS M 18 LE3 T 1 2 3 372 372 MS F 17 GT3 T 2 2 1 373 373 MS F 17 GT3 T 1 2 1 374 374 MS F 18 LE3 T 4 4 2 375 375 MS F 18 GT3 T 1 1 4 376 376 MS F 20 GT3 T 4 2 2 377 377 MS F 18 LE3 T 4 4 1 378 378 MS F 18 GT3 T 3 3 1 379 379 MS F 17 GT3 T 3 1 1 380 380 MS M 18 GT3 T 4 4 1 381 381 MS M 18 GT3 T 2 1 2 382 382 MS M 17 GT3 T 2 3 2 383 383 MS M 19 GT3 T 1 1 2 384 384 MS M 18 GT3 T 4 2 2 385 385 MS F 18 GT3 T 2 2 2 386 386 MS F 18 GT3 T 4 4 3 387 387 MS F 19 GT3 T 2 3 1 388 388 MS F 18 LE3 T 3 1 1 389 389 MS F 18 GT3 T 1 1 2 390 390 MS M 20 LE3 A 2 2 1 391 391 MS M 17 LE3 T 3 1 2 392 392 MS M 21 GT3 T 1 1 1 393 393 MS M 18 LE3 T 3 2 3 394 394 MS M 19 LE3 T 1 1 1 failures ... goout Dalc Walc health absences G1 G2 G3 location \ 0 0 ... 4 1 1 3 6 5 6 6 Urban 1 0 ... 3 1 1 3 4 5 5 6 Urban 2 3 ... 2 2 3 3 10 7 8 10 Urban 3 0 ... 2 1 1 5 2 15 14 15 Urban 4 0 ... 2 1 2 5 4 6 10 10 Urban 5 0 ... 2 1 2 5 10 15 15 15 Urban 6 0 ... 4 1 1 3 0 12 12 11 Urban 7 0 ... 4 1 1 1 6 6 5 6 Urban 8 0 ... 2 1 1 1 0 16 18 19 Urban 9 0 ... 1 1 1 5 0 14 15 15 Urban 10 0 ... 3 1 2 2 0 10 8 9 Urban 11 0 ... 2 1 1 4 4 10 12 12 Urban 12 0 ... 3 1 3 5 2 14 14 14 Urban 13 0 ... 3 1 2 3 2 10 10 11 Urban 14 0 ... 2 1 1 3 0 14 16 16 Urban 15 0 ... 4 1 2 2 4 14 14 14 Urban 16 0 ... 3 1 2 2 6 13 14 14 Urban 17 0 ... 2 1 1 4 4 8 10 10 Urban 18 3 ... 5 2 4 5 16 6 5 5 Urban 19 0 ... 3 1 3 5 4 8 10 10 Urban 20 0 ... 1 1 1 1 0 13 14 15 Urban 21 0 ... 2 1 1 5 0 12 15 15 Urban 22 0 ... 1 1 3 5 2 15 15 16 Urban 23 0 ... 4 2 4 5 0 13 13 12 Urban 24 0 ... 2 1 1 5 2 10 9 8 Rural 25 2 ... 2 1 3 5 14 6 9 8 Urban 26 0 ... 2 1 2 5 2 12 12 11 Urban 27 0 ... 4 2 4 1 4 15 16 15 Urban 28 0 ... 3 1 1 5 4 11 11 11 Urban 29 0 ... 5 5 5 5 16 10 12 11 Urban .. ... ... ... ... ... ... ... .. .. .. ... 365 0 ... 4 2 4 3 4 10 10 10 Rural 366 0 ... 2 2 2 5 0 13 13 13 Urban 367 1 ... 1 1 2 1 0 7 6 0 Rural 368 0 ... 3 1 2 4 0 11 10 10 Urban 369 0 ... 2 4 2 5 10 14 12 11 Rural 370 2 ... 2 1 1 3 4 7 7 9 Urban 371 0 ... 3 2 3 3 3 14 12 12 Rural 372 0 ... 3 1 1 3 8 13 11 11 Urban 373 0 ... 5 1 3 1 14 6 5 5 Rural 374 0 ... 4 1 1 1 0 19 18 19 Rural 375 0 ... 2 1 2 4 2 8 8 10 Rural 376 2 ... 3 1 1 3 4 15 14 15 Urban 377 0 ... 3 3 4 2 4 8 9 10 Rural 378 0 ... 3 1 2 1 0 15 15 15 Urban 379 0 ... 4 2 3 1 17 10 10 10 Rural 380 0 ... 4 1 4 2 4 15 14 14 Urban 381 0 ... 3 1 3 5 5 7 6 7 Rural 382 0 ... 3 1 1 3 2 11 11 10 Urban 383 1 ... 2 1 3 5 0 6 5 0 Rural 384 1 ... 3 4 3 3 14 6 5 5 Rural 385 0 ... 3 1 3 4 2 10 9 10 Rural 386 0 ... 3 2 2 5 7 6 5 6 Rural 387 1 ... 2 1 2 5 0 7 5 0 Rural 388 0 ... 4 1 1 1 0 7 9 8 Urban 389 1 ... 1 1 1 5 0 6 5 0 Urban 390 2 ... 4 4 5 4 11 9 9 9 Urban 391 0 ... 5 3 4 2 3 14 16 16 Urban 392 3 ... 3 3 3 3 3 10 8 7 Rural 393 0 ... 1 3 4 5 0 11 12 10 Rural 394 0 ... 3 3 3 5 5 8 9 9 Urban study_time 0 2 to 5 hours 1 2 to 5 hours 2 2 to 5 hours 3 5 to 10 hours 4 2 to 5 hours 5 2 to 5 hours 6 2 to 5 hours 7 2 to 5 hours 8 2 to 5 hours 9 2 to 5 hours 10 2 to 5 hours 11 5 to 10 hours 12 <2 hours 13 2 to 5 hours 14 5 to 10 hours 15 <2 hours 16 5 to 10 hours 17 2 to 5 hours 18 <2 hours 19 <2 hours 20 2 to 5 hours 21 <2 hours 22 2 to 5 hours 23 2 to 5 hours 24 5 to 10 hours 25 <2 hours 26 <2 hours 27 <2 hours 28 2 to 5 hours 29 2 to 5 hours .. ... 365 2 to 5 hours 366 5 to 10 hours 367 <2 hours 368 <2 hours 369 2 to 5 hours 370 2 to 5 hours 371 <2 hours 372 5 to 10 hours 373 <2 hours 374 5 to 10 hours 375 5 to 10 hours 376 5 to 10 hours 377 2 to 5 hours 378 2 to 5 hours 379 2 to 5 hours 380 2 to 5 hours 381 <2 hours 382 2 to 5 hours 383 <2 hours 384 <2 hours 385 5 to 10 hours 386 <2 hours 387 5 to 10 hours 388 2 to 5 hours 389 2 to 5 hours 390 2 to 5 hours 391 <2 hours 392 <2 hours 393 <2 hours 394 <2 hours [395 rows x 30 columns]
# Adjust to add subplots based on school support sns.relplot(x="G1", y="G3", data=student_data, kind="scatter", col = "schoolsup", col_order=["yes", "no"]) # Show plot plt.show()
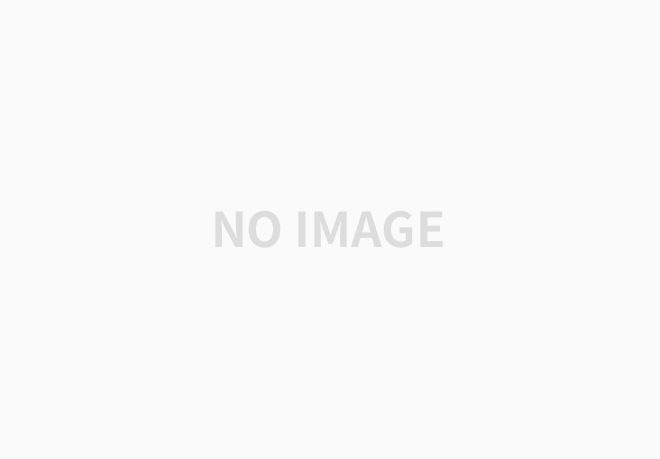
# Adjust further to add subplots based on family support sns.relplot(x="G1", y="G3", data=student_data, kind="scatter", col="schoolsup", col_order=["yes", "no"], row="famsup", row_order=["yes", "no"]) # Show plot plt.show()
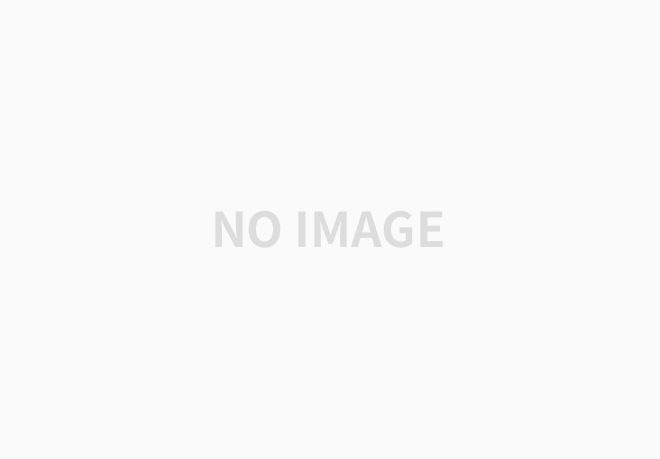
All the Contents are from DataCamp
Learn R, Python & Data Science Online
www.datacamp.com